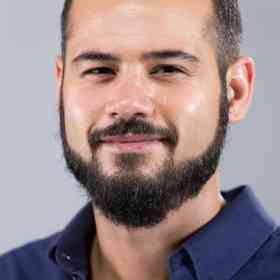
Taylor M. Oshan (University of Maryland)
Generalized Additive Spatial Smoothing (GASS)
The geographical context of individuals, households, or places of interest is often conceptualized using ‘neighborhoods’ that encapsulate the characteristics of the surrounding environment. ‘Neighborhood effects’ capture the relationship between an outcome and one or more variables defined within the neighborhood around the outcome. The definition of neighborhoods is therefore critical for accurately measuring neighborhood effects in a wide variety of applications across disciplines, such as sociology, epidemiology, economics, urban planning, and criminology. Though administrative boundaries are sometimes conveniently used as neighborhoods, they may not actually align with neighborhoods as they are experienced and are limited in their ability to represent their inherently ‘fuzzy’ nature. Another strategy, sometimes referred to as ‘egohoods’, employs potentially overlapping buffers and distance-weighted smoothing functions around each observation in order to construct fuzzy, idiosyncratic neighborhoods. However, it is often not clear how to parameterize egohoods, such as selecting the appropriate buffer size or smoothing function, and the same parameters (i.e., spatial scale) are assumed to apply for each relationship in a model. This paper introduces a new methodology called generalized additive spatial smoothing (GASS) that provides a flexible multiscale smoothing framework for simultaneously selecting variable-specific neighborhoods and modeling neighborhood effects.